The Essential Guide to Medical Data Annotation
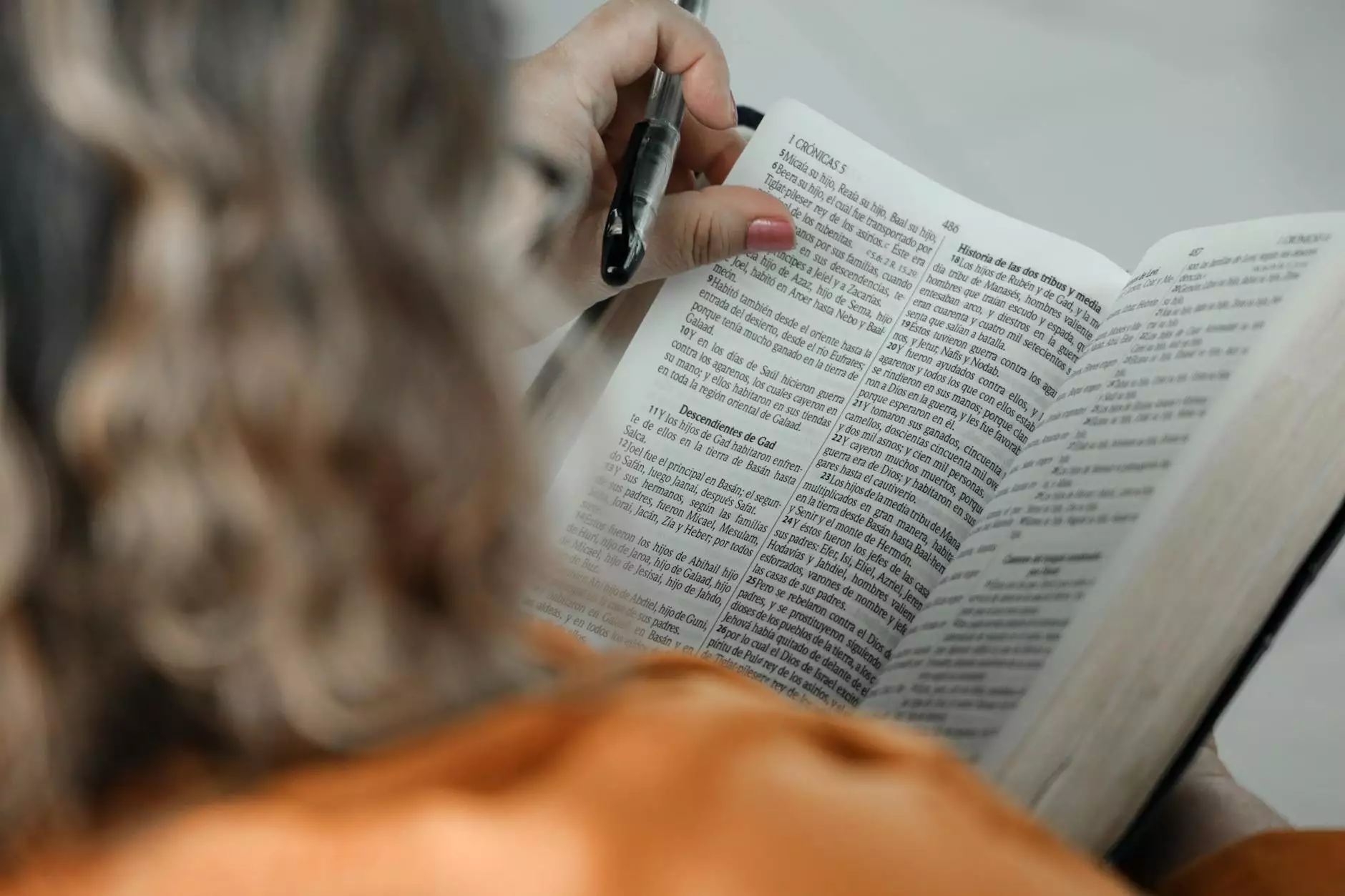
Medical data annotation is at the forefront of transforming healthcare and enhancing the efficacy of artificial intelligence (AI) in medicine. As healthcare evolves with technology, the demand for accurately labeled medical data grows, paving the way for improved diagnostics, treatment plans, and patient outcomes. In this comprehensive guide, we will delve into the key aspects of medical data annotation, its significance, methodologies, and future directions.
Understanding Medical Data Annotation
Medical data annotation involves the process of labeling various types of medical data that encompass images, text, and recordings. This labeling is crucial for training machine learning models that drive AI in the healthcare sector. Accurate annotations allow AI systems to learn and identify patterns, which can lead to better predictions in areas such as disease detection, treatment personalization, and patient care efficiency.
The Importance of Medical Data Annotation
The importance of medical data annotation cannot be overstated. Here are some compelling reasons why precise annotations are vital:
- Enhanced Diagnostic Accuracy: Properly annotated data aids in training algorithms that can identify diseases with high precision, reducing misdiagnoses.
- Streamlined Patient Care: Annotated records help in quickly retrieving patient histories and treatment plans, allowing for better healthcare delivery.
- Improved Research and Development: Accurate labeled data supports clinical research initiatives and the development of new treatments.
- Regulatory Compliance: Annotation ensures that medical datasets meet regulatory standards, crucial for approvals in healthcare AI.
Types of Medical Data That Require Annotation
There are several types of medical data that necessitate annotation, each serving unique purposes within the healthcare ecosystem. Here are the primary categories:
1. Medical Imaging
Medical imaging encompasses a broad range of diagnostic techniques including MRI, CT scans, and X-rays. Annotating these images requires identifying areas of interest, such as tumors or abnormalities. The common practices include:
- Image Segmentation: Dividing an image into segments to simplify its analysis.
- Bounding Box Annotation: Drawing rectangular boxes around specific regions of interest.
- Landmark Annotation: Marking precise points on anatomical structures.
2. Clinical Text Data
Clinical texts, such as electronic health records (EHRs), require annotation for various elements like symptoms, procedures, and diagnoses. Key annotation techniques include:
- Named Entity Recognition (NER): Identifying and classifying entities within the text.
- Text Classification: Categorizing text into predefined classes.
- Relation Extraction: Finding relationships between different entities in the text.
3. Audio and Voice Data
With the rise of telemedicine and patient monitoring, audio recordings of consultations also need to be annotated. This includes:
- Transcription: Converting audio speech into written text.
- Sentiment Analysis: Determining the sentiment expressed in conversations.
Challenges in Medical Data Annotation
While medical data annotation holds immense potential, it is not without challenges:
- Data Privacy: Ensuring patient confidentiality while annotating sensitive data.
- Quality Control: Maintaining high accuracy in annotations, which can significantly affect AI outcomes.
- High Volume of Data: The sheer abundance of medical data can overwhelm annotation processes.
- Complex Medical Terminology: The necessity for specialized knowledge to navigate medical jargon effectively.
Best Practices for Effective Medical Data Annotation
To overcome challenges and ensure high-quality annotations, several best practices should be adopted:
1. Employing Expert Annotators
Utilize professionals with medical knowledge, such as doctors or radiologists, to ensure that annotations are precise and contextually relevant.
2. Utilizing Annotation Tools and Software
Invest in advanced annotation tools that facilitate collaborative efforts, streamline workflows, and enhance accuracy through automation.
3. Implementing Regular Quality Checks
Establish a rigorous quality assurance process, including multiple layers of oversight and validation, to uphold annotation standards.
4. Continuous Training for Annotators
Provide ongoing education for annotators to keep them updated on the latest medical developments and annotation techniques.
Future Trends in Medical Data Annotation
As technology continues to evolve, the field of medical data annotation will witness remarkable trends that will further enhance its effectiveness:
1. Artificial Intelligence and Automation
AI itself plays a dual role: It is a beneficiary of annotated data, but it is also becoming a tool to assist in annotation. Automation can expedite the process, reduce human error, and even execute bulk annotations based on learned patterns.
2. Crowdsourcing Annotation
Leveraging crowdsourcing can significantly increase the volume of annotated data. This method allows a diverse set of contributors, although it requires careful management to ensure quality.
3. Integration with Blockchain Technology
Blockchain can provide a secure and transparent method for tracking data annotation processes, ensuring ethical practices in data handling and maintaining data integrity.
4. Expanding Applicability Beyond Healthcare
While currently focused on the healthcare sector, the methodologies and technologies developed for medical data annotation may also find applications in fields such as pharmaceutical research and public health.
Conclusion
In a world increasingly reliant on data-driven decisions, the role of medical data annotation enhances collaboration between healthcare professionals and AI systems. This essential process not only underpins advancements in diagnostics and treatment strategies but also shapes the future of patient care. As the industry continues to innovate, staying ahead in the practices of medical data annotation will be paramount for healthcare providers aiming for excellence in patient outcomes.
For more information and expert services in medical data annotation, visiting websites like keymakr.com can be incredibly beneficial. Together, let us work toward a future where medical data is not only abundant but also insightful and actionable.